Scroll Down
Scroll Down
Scroll Down
The future of the city lies in its veins
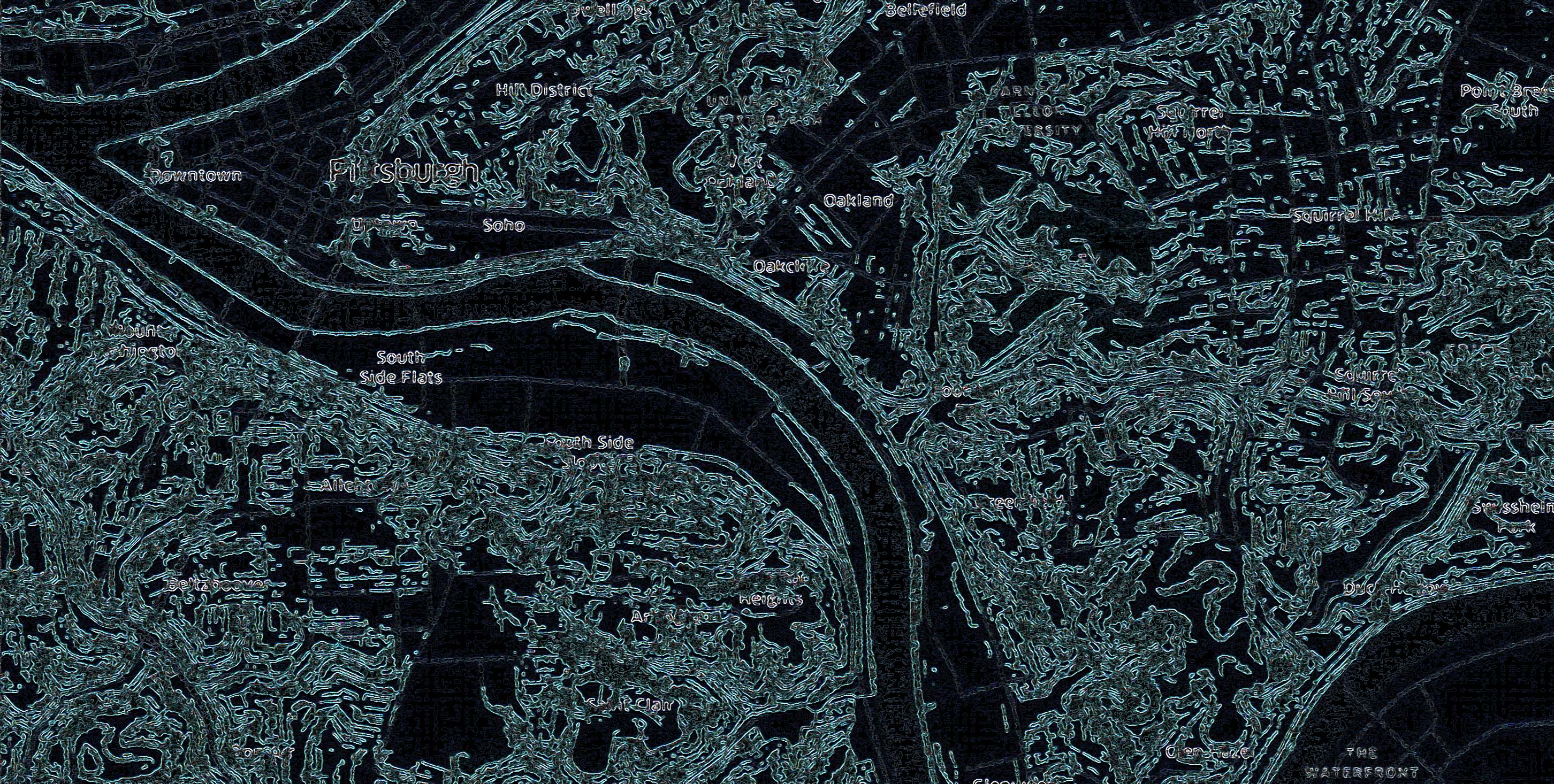
The city is back in BETA
The purpose of this work is to examine current stormwater infrastructure systems and examine the efficacy of implementing an operations management program for a resilient system. This study investigates the current and future of stormwater infrastructure management through a case study of the Gates building at Carnegie Mellon University. A critical analysis of the current stormwater infrastructure systems is performed via GIS modeling, supply and demand modeling, statistics, and predictive modeling serves as a base platform for an operations management model. With the integration of existing IoT technology, we sought to understand how a resilient stormwater model could emerge through the collaboration of existing data, meteorology predictive studies and current stormwater management strategies.
Done in collaboration with : Alex Lewis
Support and guidance from the Carnegie Mellon Civil and Environmental Engineering Department
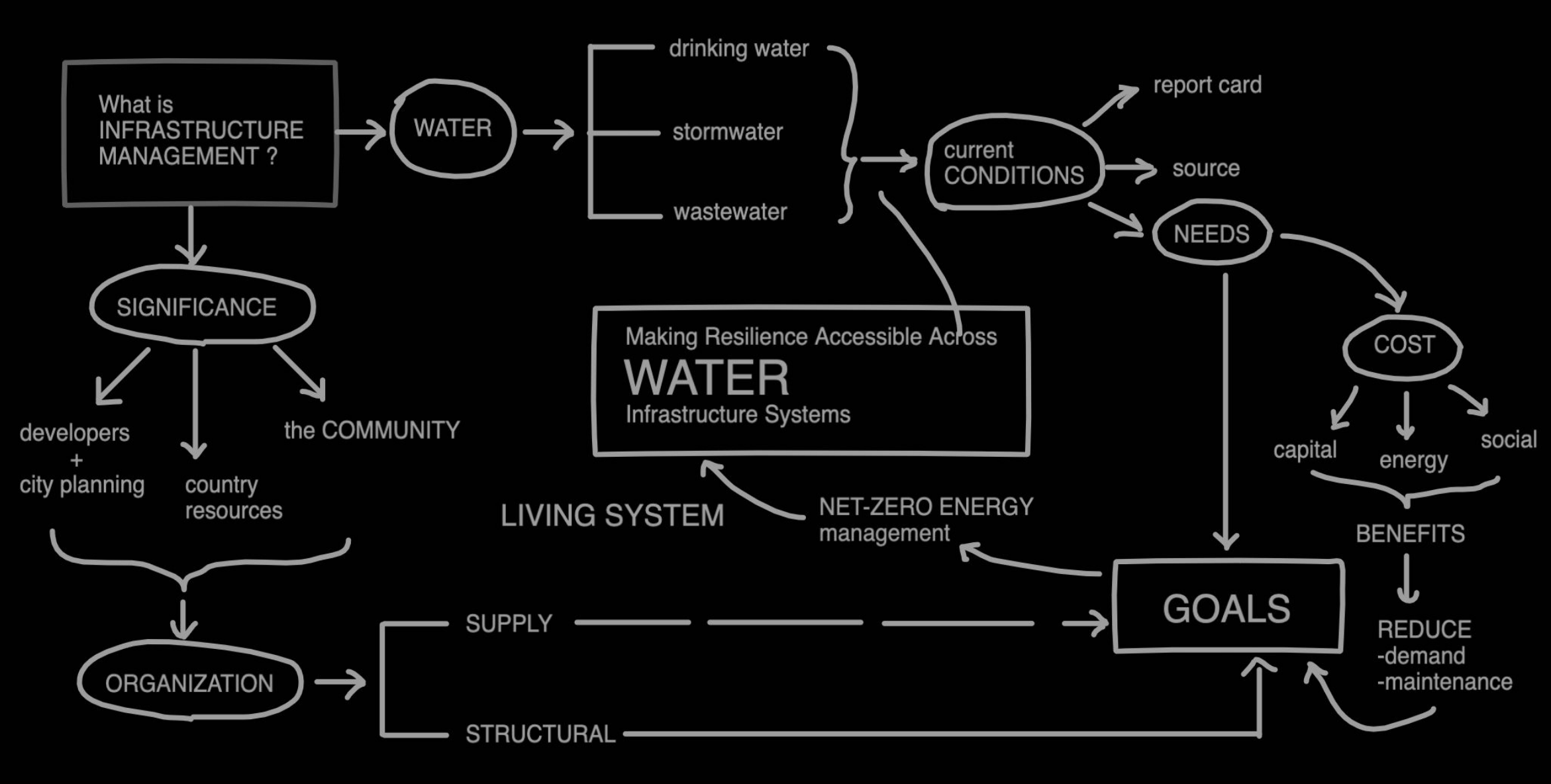
Phase One : Water
The average human in the United States uses 80-100 gallons of water per day performing a myriad of tasks (Perlman 2016). That 80-100 gallons of water totals to 29,200-36,500 gallons of water per year used by one person. In the city of Pittsburgh, a population of approximately 300,000 people have a water demand over 11 billion gallons of water each year (Census 2017). The problem is : our water systems are failing. An emphasis on pre-existing infrastructure maintenance continues to decrease and in the face of climate change, that is only the beginning of our problems. There are many areas in desperate need of improvement in city infrastructure and I am interested in exploring each sector in greater depth. Water managment seves as only the first phase in the process.
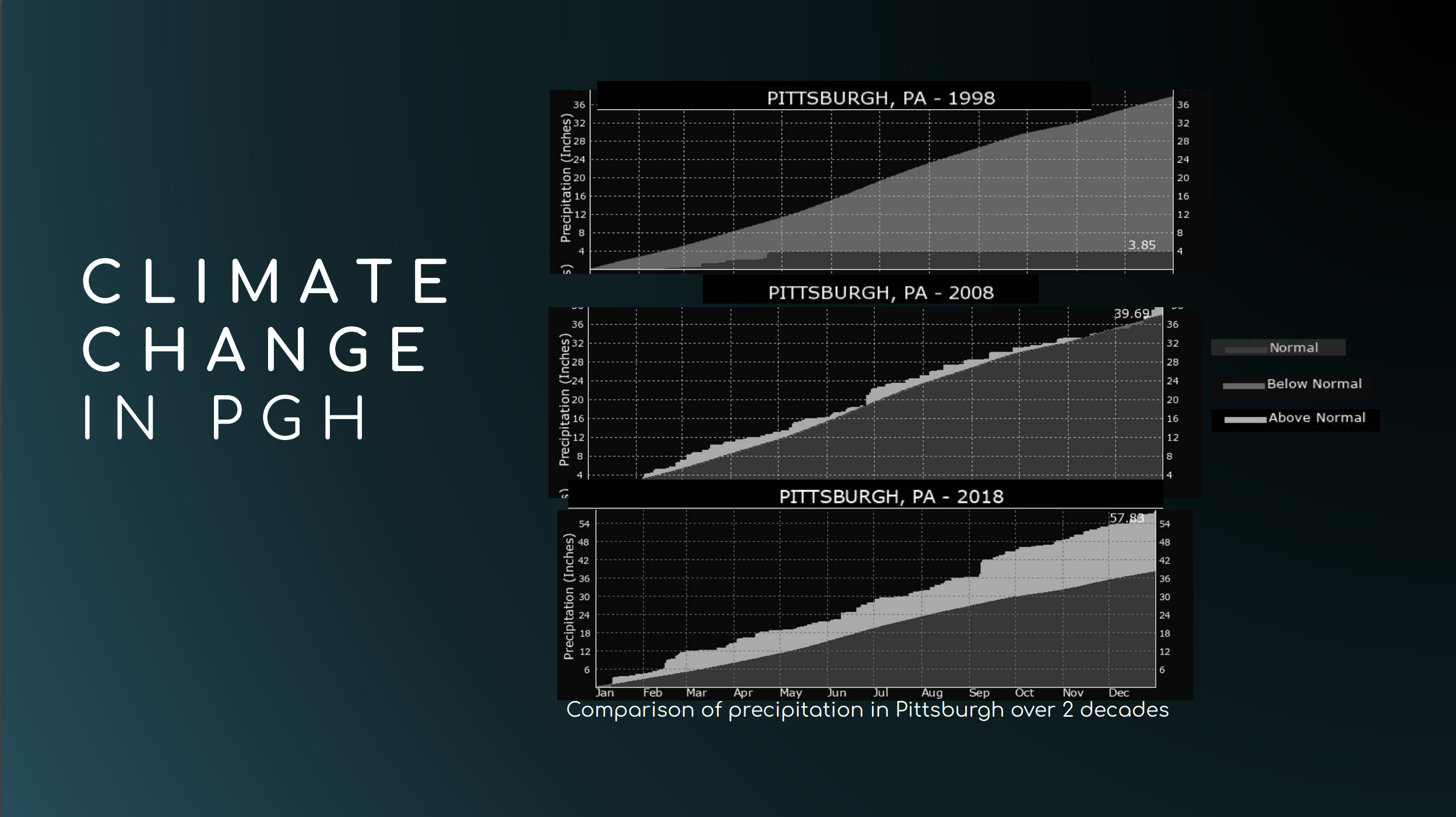
Our Proposal
Carbon emissions and methane levels in the air have a direct influence on density and pressure of the air, temperature of the air and the associated likeliness of cloud formations. Such presence of emissions in the air makes it increasingly difficult to understand how much rainfall will occur and on what basis of natural patterns as rainfall patterns is becoming increasingly less associated with natural cycles as it is with climate change impacts. NASA is currently looking to capture and monitor the air quality around major cities utilizing data sources from 5 major covariance towers across Alaska. If similar towers were integrated in proximity to major cities, the data collected could be integrated into a machine learning platform that reads the binary states of methane and carbon flux patterns and outputs a prediction based on the mixing rate of the two with the air to determine whether rain will occur and if so, based on the degree of mixing rate, the predicted amount. Relying on the prediction of rain based on a city’s ambient pollution would help to mitigate inaccuracies in weather prediction and also given more time to react and prepare for oncoming rainfall. The current model we worked with for creating such machine learning model was based in Weka. The figure below shows the base binary predictions of different flux patterns. These patterns are for an entire year, however, the data can be optimized for daily predictions as well as be fitted to user demand cirves.
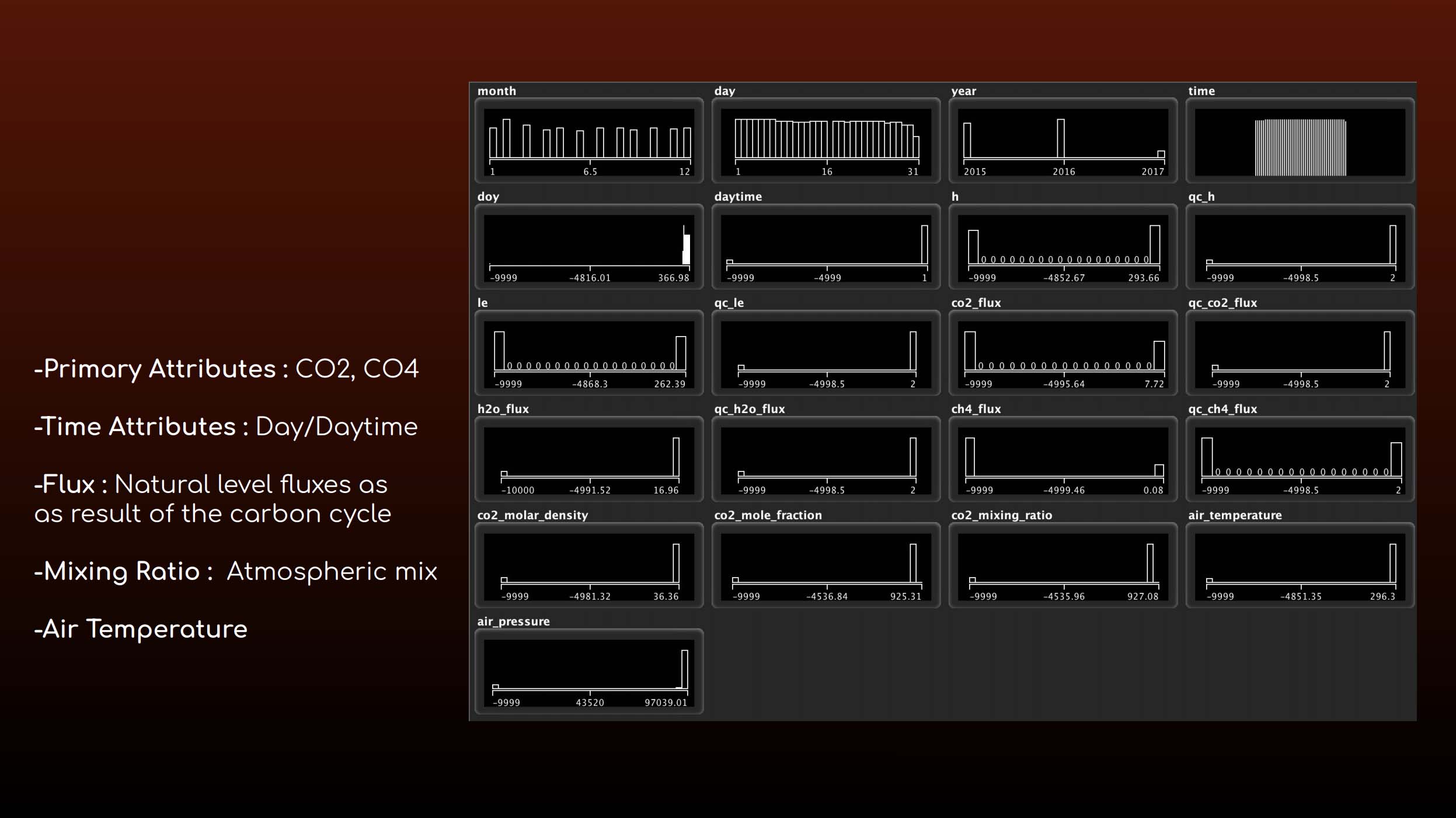
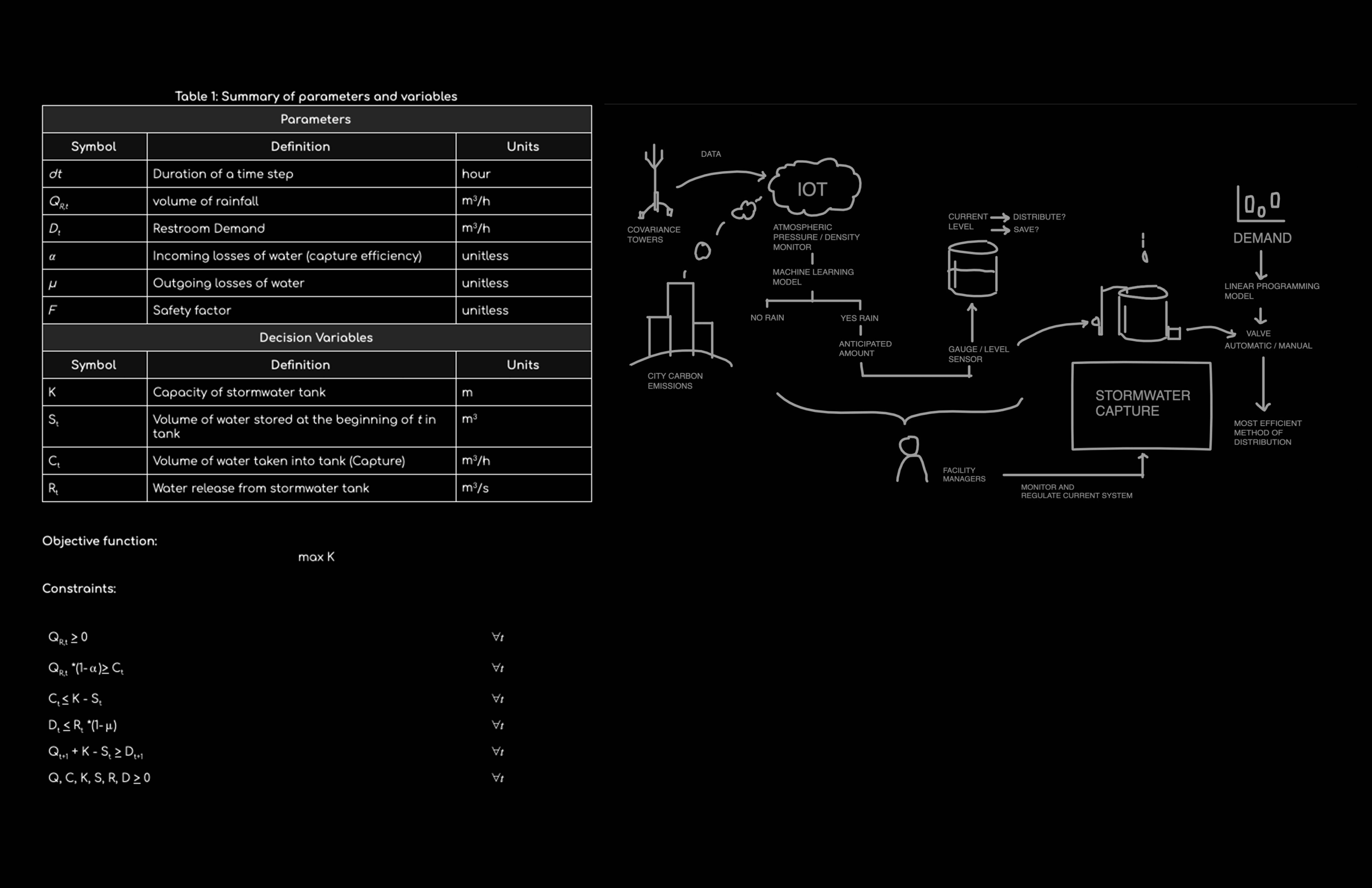